AI + Experience: Unlocking the Future of Capital Markets Infrastructure
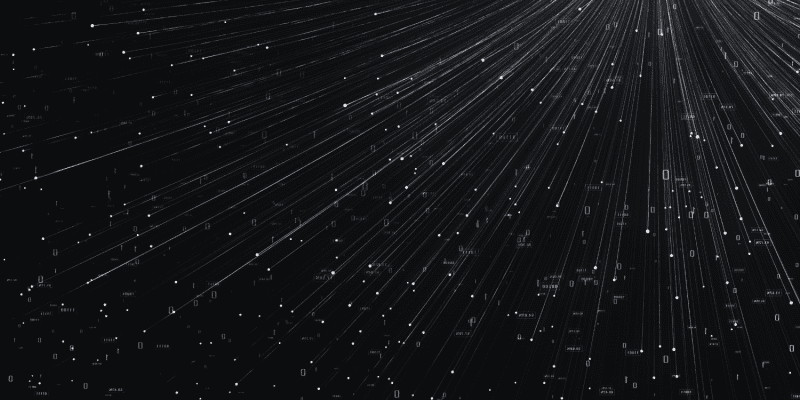
Leveraging AI to enhance human expertise in Capital Markets is key to ensuring efficiency, compliance, and innovation without losing institutional knowledge.
Artificial Intelligence (AI) has the potential to transform Capital Markets infrastructure operations, powering tools to scale expertise, automate workflows, and enhance decision-making. However, AI alone is not enough, and whilst it provides a platform for scaling knowledge, it isn’t a replacement for expertise and the real power lies in the fusion of AI-driven insights with human experience.
As financial markets start to adopt agentic workflows to improve productivity, the challenge is ensuring AI enhances, rather than replaces, the deep domain knowledge that underpins critical market operations. This balance between automation and human oversight is the key to unlocking a new era of efficiency, agility, and innovation in Capital Markets infrastructure, whilst ensuring we don’t create a future experience vacuum.
AI as an Accelerator, Not a Replacement
AI has proven its ability to process vast amounts of unstructured data, extract insights, and automate repetitive tasks. In Capital Markets, this means AI can codify complex documents—such as exchange connectivity guides, API specifications, and market data policies —into structured, actionable data. This structured intelligence can then drive automated workflows, reducing the manual effort required to manage market access, trading infrastructure, and data services.
However, AI is not a substitute for human experience. Capital Markets infrastructure is built on decades of institutional knowledge, nuanced regulatory frameworks, and intricate market structures and data sets. Whilst AI can enhance efficiency, it requires human oversight to ensure accuracy, contextual relevance, and compliance. The most effective platforms will be those that allow firms to embed their experience within AI-enhanced workflows, ensuring automation aligns with real-world market operations and accelerating existing processes rather than replacing them.
Embedding Experience in AI-Driven Workflows
A successful agentic AI strategy incorporates expertise into the platform by designing systems that allow users to customise workflows, define decision-making parameters, and refine AI-generated outputs. Successful platforms will provide:
- Customisable AI Workflows – Firms must be able to tailor AI-driven processes to reflect their specific operational model, regulatory requirements, and market nuances.
- Human-in-the-Loop Oversight – AI should handle the 80% of routine tasks, allowing human experts to focus on the 20% of critical, high-value decisions and exceptions.
- Contextual Intelligence – Agents and models need the right context to make decisions, for example, leveraging structured service catalogues that define the ontology of market infrastructure, ensuring AI-generated insights are relevant and actionable.
By embedding expertise into agentic workflows, firms can create a hybrid model where automation accelerates routine tasks while human experience ensures accuracy, compliance, and strategic alignment.
Avoiding the ‘Experience Gap’ in Capital Markets
As agentic AI adoption accelerates, firms must guard against the risk of an ‘experience gap’ – a scenario where over-reliance on automation erodes institutional knowledge. In Capital Markets, where expertise in exchange connectivity, trading infrastructure, and market data services remains niche, this risk is particularly acute.
The skills shortage challenge is something that’s regularly discussed at industry events, to understand how we can inspire the next generation of exchange connectivity and market data experts. This is particularly important given some of the aging technologies and platforms still in operation today, and the limited expertise to operate them.
Whilst agentic AI can scale expertise and refocus critical resources on more value-add business activities, it’s crucial we don’t create a longer term ‘experience vacuum’ as we automate these manual, repetitive tasks.
We need to take a thoughtful, pragmatic approach to adopting this technology to support critical trading and data infrastructure and ensure we don’t exasperate the experience gap as we automate and streamline processes. This is core to how we’re developing our platform, as we focus on these key principles:
- Explainability and Control: The 80/20 rule highlights the need for AI to automate routine tasks whilst ensuring human oversight for critical decisions. AI should offer clear audit trails and transparent reasoning to enhance efficiency and accountability, building trust and reliability.
- Transparency and Demarcation: Clearly distinguish between human and AI roles, ensuring everyone knows when and how AI is used. Transparency builds trust by clarifying AI involvement and maintaining informed, responsible use without single points of failure.
- Preserve Institutional Knowledge: AI should be used to document, codify, and share expertise, ensuring critical knowledge is an asset that is retained, accessible and constantly refined.
- Enable Agentic Workflows: AI should not just automate tasks but empower users with intelligent, actionable insights, guiding them through complex decisions while preserving human control.
- Ensure Continuous Learning – AI models should evolve based on user feedback, market changes, and regulatory updates, ensuring they remain aligned with real-world conditions.
By proactively addressing the experience gap, firms can harness AI’s power without losing the human expertise that underpins market stability and efficiency.
The Future of AI in Capital Markets Infrastructure
The future of Capital Markets operations is not AI versus human expertise – it is human experience enhanced with agentic workflows. The most successful firms will be those that strike the right balance between automation and human oversight, leveraging AI to enhance efficiency whilst embedding experience into every workflow.
As AI continues to evolve, the focus must be on building platforms that are adaptable, intelligent, and experience-driven. This means:
- Dynamic, Data-Driven Infrastructure – AI-powered platforms should enable seamless market access, automated provisioning, and intelligent decision-making.
- Composable, Modular Solutions – Firms should adopt a phased approach to AI integration, leveraging operational middleware to modernise infrastructure without the need for wholesale re-engineering.
- AI-Augmented Expertise – The goal is not to replace human expertise but to amplify it, ensuring AI serves as an enabler rather than a disruptor.
By embracing this approach, firms can unlock new levels of agility, efficiency, and innovation in a safe and scalable way – ensuring they remain competitive and compliant in an increasingly digital-first world