Garbage In, Garbage Out: The imperative for accurate data in an AI-driven world
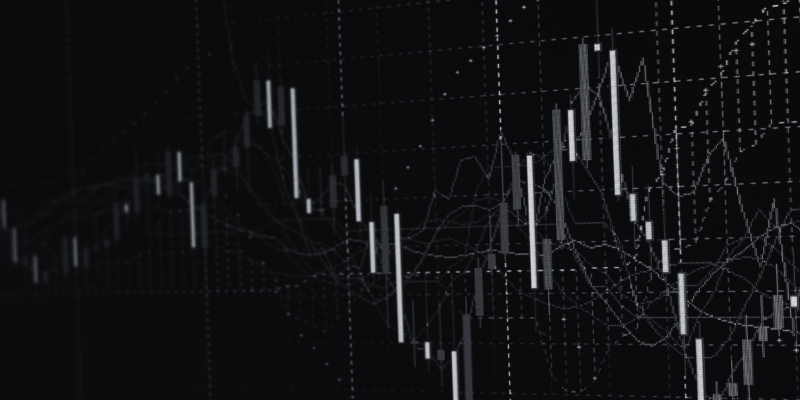
Artificial Intelligence (AI) is increasingly transforming the financial services sector, particularly in trade execution and associated transaction processing workflows. AI’s ability to quickly process very large amounts of data and derive actionable insights opens up a plethora of opportunities in the world of financial trading, especially in the context of reference data.
Artificial Intelligence (AI) is increasingly transforming the financial services sector, particularly in trade execution and associated transaction processing workflows. AI’s ability to quickly process very large amounts of data and derive actionable insights opens up a plethora of opportunities in the world of financial trading, especially in the context of reference data.
The many positives of AI applications to support “big data” processing and analysis, both to support automated, algorithmic and quant trading strategies at the front end, and other process workflows – for example, client and regulatory reporting – in middle and back-office functions are evident. However, the effectiveness and reliability of AI-driven outcomes has a huge dependency on a starting point of high quality, reliable and consistent data.
The GIGO theory – garbage in, garbage out – first mooted in the 1960s for nascent IBM computing remains as true today as it was then. The output of computing technologies, including AI (AI), machine learning (ML) and the internet of things (IoT), will only be as good as the data used to ‘feed the machine’, regardless of whether the associated program logic or mathematical model is sound. This is true of all data-driven systems and processes; precise, accurate and consistent data is essential to generate the right results and to inform the right decisions.
AI in Financial Trading
Financial trading for all asset classes and associated derivatives relies increasingly and heavily on systematised data processing to analyse and interpret patterns and behaviours and derive actionable insights that can inform trading strategies. Traders might use historical data, market trends, news feeds and multiple other sources and types of information: data, AI, and machine learning (ML) in particular, create the ability to analyse these vast datasets in real time, quickly finding patterns and trading signals that might elude human analysis. AI applications in financial trading can broadly be categorised into a few key areas:
Algorithmic Trading: AI models seek to maximise returns using historical [and real time] data to predict future price movements, executing trades automatically subject to ‘hitting’ pre-programmed rules. Algorithms can process a broad and deep range of data inputs exponentially faster than any human, enabling trading strategies that can exploit the smallest movements and market trends to maximise potential returns.
Market Sentiment:AI can scan vast amounts of unstructured data from news outlets, social media and other sources, and even trade execution ‘noise’ in electronic and trader voice channels, to gauge market sentiment and help traders understand emotional undertones in the market – as well as actual market movements.
Risk Management: AI is also used to help financial firms identify and mitigate trade execution and trade processing risks. By analysing data and market conditions, AI algorithms can forecast potential market downturns, credit risks and liquidity issues, giving traders ‘intelligence’ they can act on to adjust trading strategies. AI can also be used to support complex regulatory reporting workflows and reduce the risks of reporting errors and remediation.
Value and application of Reference Data in Financial Trading
Reference data feeds trading systems, algorithms and transaction processing workflows. As the essential information that describes the “what, where and how” of the instruments being traded it forms the bedrock of all trading activities. Reference data embraces things such as security identifiers (e.g. ISINs and CUSIPs), venue data (exchange), counterparty IDs, exchange rates, volume and open interest data, trading and holiday dates – pretty much everything, in fact, bar the price itself (market data).
Since around 70% of all data associated with trading is estimated to be reference data, its accuracy is critical to the smooth functioning of trading systems and operations. Without validated, consistent and accurate data, even the most sophisticated AI models would be unable to produce reliable outcomes, impairing trading decisions and impacting returns (and profitability).
As well as being able to integrate and aggregate data and information from multiple sources, AI can also help with the detection of data anomalies and missing data points – flagging potential issues before they disrupt trading activities – or to assure the accuracy of mandated transaction reports.
While AI has the potential to transform the financial trading landscape, accurate, quality data will continue to be critical to ensure the best outcomes. “Garbage in, garbage out” remains an abiding principle and reminds us that even the most advanced AI models cannot perform optimally with poor quality data. As AI innovation continues to evolve with respect to deep learning, natural language processing, quantum computing and the like, the imperative for clean, well-managed data will only become more crucial.
Ensuring data accuracy is not just a technical necessity but a strategic priority for firms looking to stay ahead in the rapidly advancing trading landscape. By prioritising data quality, firms can unlock the full potential of AI-driven trading strategies and transaction processing and maintain a competitive edge.