How to harness small data and GenAI in Financial Services: The power of people, precision, and automation.
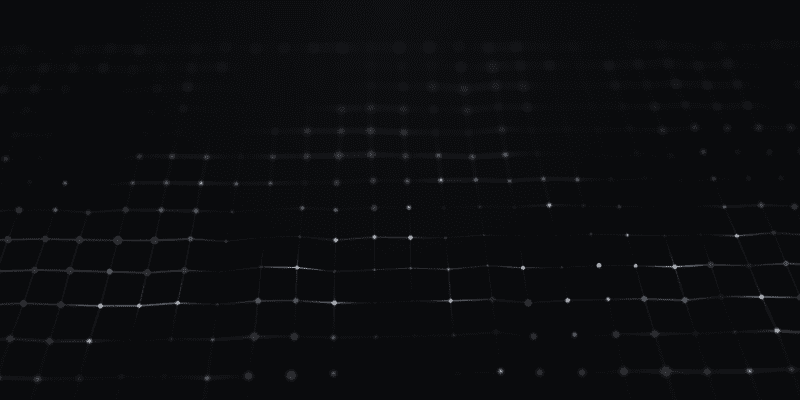
In the second blog, in our series on GenAI and LLMs (Large Language Models) in financial services, we assess the importance of human interaction and smaller data in achieving the best results.
Why small data sets are a secret weapon for big banks
Reducing costs and being first to market can make all the difference for global financial services institutions.
In this blog, we’ll explore the ‘big’ debate on big data, explore why sometimes smaller is smarter, and reveal how keeping humans in the loop creates a powerful trio for cost-effective, robust, reliable, and accurate solutions.
The debate is a strategic one. The winning argument has important implications for overall accuracy and performance. Today, much of the industry buzz is focused on big data but there are industry experts who promote and champion the benefits of small data sets, because when coupled with the integration of large language models (LLMs) – this approach offers a game-changing advantage for financial services firms looking to enhance their data extraction and automation processes.
Crucially, human expertise remains an indispensable element of this powerful trio.
Why has big data dominated the conversation in financial services?
Big data refers to data sets that are so large and complex that they can’t be processed by humans alone. While the attraction of big data is in its promise of deeper and more comprehensive insights into consumer behaviour and business trends, it has its own set of challenges — such as complexity, high costs, and lengthy training times — which can be unmanageable for many organisations.
A 2023 Gartner survey showed that almost 47% of employees struggled to even find relevant data in documents needed to perform their jobs. It’s more important than ever for businesses, especially within financial services, to drive employee operational and productivity gains.
So what is the alternative to big data?
How small data can deliver big results for leading banks
Rather than just giving a broad overview (which big data often does), analysing small data allows innovation teams to pinpoint the specific information needed to tackle organisational issues.
This targeted approach leads to better results, increasing buy-in across the organisation and helping it become truly data-driven.
There is a compelling argument for the power of small data, specifically targeted to an organisation’s needs, offering a few key advantages:
Higher efficiency: Small data is easier to manage and process, requiring less computational power and shorter training periods.
Better accuracy: With less noise and more relevant data points, models trained on small data often yield more accurate results.
More accessibility: Smaller entities can gain valuable insights without needing to accumulate vast amounts of data, democratising access to advanced analytics.
Focused training: LLMs trained on small data sets are more focused and aligned with the specific business objectives, improving the quality and relevance of the outputs.
Consider, for instance, a financial institution looking to improve its loan approval process. By using small data, the organisation can quickly train models to identify key risk factors and streamline the approval process with high accuracy, even with limited historical data.
For highly specific applications like intelligent document processing (IDP) or automated insurance underwriting, small data is the ideal choice. And by combining small data sets with LLMs, financial services firms can vastly improve their data extraction and automation processes.
Generative insights can revolutionise data extraction: How does it work?
As we saw in the first blog, Intelligent document processing (IDP) is a solution that helps companies capture data from structured, semi-structured, and unstructured documents and efficiently automate data extraction and analysis by using GenAI, machine learning, and intelligent automation to sort, organise, extract meaningful data, and validate the extracted data to enhance precision and improve workflow efficiency.
IDP specialists who use LLMs in a compliant, cost-effective, and industry-specific manner provide the best solutions for enterprises aiming to unlock data from documents with advanced AI.
Developing a comprehensive model governance and human exception handling framework is vital to ensure maximum accuracy and value, while minimising model risk in regulatory compliance automation.
And when integrated with LLMs, we get what we’re calling generative insights. It’s a way of enhancing the data extraction modelling process in several transformative ways:
Better data extraction: IDP platforms can handle diverse document types (whether that’s annual reports, board papers, meeting minutes, huge Excel files, or financial regulations) and extract relevant information with greater precision and speed.
More streamlined processes: Automation reduces manual effort, freeing up human resources for more strategic tasks, leading to higher productivity, less turnover, and happier colleagues.
Enhanced adaptability: IDP technology adapts to different use cases, from regulatory compliance to customer service, and offers tailored solutions for each.
For example, a financial services firm can use generative insights to automate the extraction of key financial metrics from annual reports, significantly reducing the time and effort required for manual processing.
Not just a ‘black box’: Human expertise is still crucial in AI-driven data extraction
You can maximise the performance and accuracy of your AI systems by adopting a human-in-the-loop (HITL) approach. In this scenario, AI handles the heavy lift while human experts review and refine the outputs. This ensures the models align with business goals as well as regulatory standards.
Human expertise is essential for oversight, quality control, and ethical considerations. Let’s take a look at each:
Quality control: People ensure that the data extracted by AI models is accurate and contextually relevant. It is important to have people as part of this process to ensure exacting quality standards are met.
Training and refining models: Domain experts refine AI models, continuously improving their performance. In addition to this, off-the-shelf IDP and ‘machine vision’ or OCR (Optical Character Recognition) platforms, these days, are engineered to allow easy integration model training.
Ethical decision-making: Human judgement is critical in making the correct decisions about data use and AI deployment. This is something that can’t be left to machines alone.
Manual data and document review is slow, error-prone, and expensive. Ideally, technology would achieve 100% data accuracy without any manual intervention throughout the process.
However, AI enhances the role of people in the data extraction process by eliminating the tedious task of copying and pasting data from one document to another, thereby freeing up valuable time for higher-value tasks.
By adding human expertise at a critical point in the process, we can maximise the performance and accuracy of the system.
The combination of small data, GenAI, and human expertise creates a powerful synergy
When it comes to data extraction modelling, the combination of small data, GenAI, and human expertise is an unbeatable combination.
By embracing the power of small data, GenAI, and human expertise, financial services firms can transform their data extraction and automation processes, achieving greater efficiency, accuracy, and strategic insight.