Unlocking the power of AI in Financial Services: A freshman’s guide to Extractive vs. Generative AI
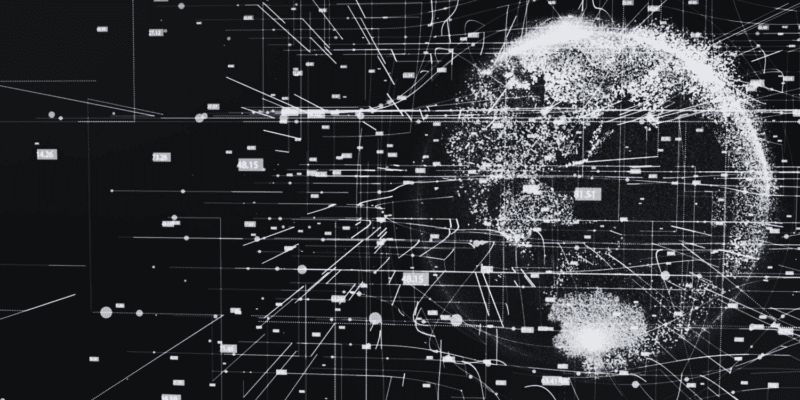
In this new series, looking at artificial intelligence in financial services, we assess the roles that Extractive and Generative AI play in your tech stack and how each measures up to the job of intelligent document processing.
How can financial institutions leverage AI to drive innovation and efficiency?
How can a global bank with 100,000 plus employees, in 65 markets, and sitting on a library of a billion documents take advantage of the most influential technology breakthrough since the birth of the Internet?
More importantly, how can you do it reliably, repeatedly, cost-effectively, and – in a systemically important sector – securely?
A recent EY-Parthenon research paper said that while banks see the transformative value of GenAI, they’re prioritising back-office automation for initial deployments.
This is a sensible approach, having strong governance models and controls in place is vital for addressing the range of risks, like data privacy, biased outcomes, and regulatory oversight.
That being said, large institutions will also need to adopt more of a start-up mentality when it comes to innovation, particularly when looking at AI solutions.
Being quick to adapt and faster to learn means innovation thrives in your organisation and this isn’t only down to innovation teams; solving real pain points where they’re pinching takes the whole enchilada.
This is about the collective back office functions collaborating and coordinating between themselves, because the benefits from a robust extractive AI solution, underpinned with an intuitive generative AI will bring big operational and productivity benefits to regulatory compliance functions, risk and control elements, legal departments – even business facing teams. So, how do you do it?
Strong intelligent document processing (IDP) should complement your natural language processing (NLP) tools
Intelligent document processing (IDP) is a solution that helps companies capture data from structured, semi-structured, and unstructured documents and efficiently automate data extraction and analysis by using GenAI, machine learning, and intelligent automation to sort, organise, extract meaningful data, and validate the extracted data to enhance precision and improve workflow efficiency.
Success with AI is perceived as difficult when it doesn’t need to be. New platforms give innovation teams the freedom and safety to test out a range of unique use cases in sandboxes (safe virtual testing grounds) without any money or time wasted and public cloud platforms now offer a range of off-the-shelf, point-and-click AI services.
There’s value in running POCs through these platforms to help validate solutions securely in your own private cloud environment before taking more formal next steps with key internal stakeholders. However, it is important to consider where and how your data is being used, for example, will it be used to train the underlying model, will it be shared with a third party, etc?
It’s also very important to understand what the hyper-specific pain points are that new tech could address within large institutions.
Assessing use case value and risk to inform a longer-term roadmap can be time-consuming and difficult to achieve but also helps in creating frameworks for use case development. With the right platform, you’re able to test use cases safely, securely, and quickly.
Understanding, in detail, from the teams themselves what their most pressing needs are can also lead to big productivity gains, as well as making the people doing manual processing or administratively heavy tasks feel more empowered, and allow them to concentrate on higher-value strategic tasks.
JP Morgan expects AI to save employees an average of 2-4 hours a day on repetitive, joyless tasks. So much so that the investment in the technology pays for itself. That’s a win-win.
How to successfully navigate regulatory risks to take advantage of outsized opportunities
Global financial institutions are unique. Their structure, regulation, risk management, and governance have been designed to ensure data integrity and protection of each entity and function. This can cause problems when trying to implement even the most efficient, effective, and elementary solutions.
Finding a software development partner with experience and expertise honed in financial services is a viable option but they will also need to understand the unique challenges of working with this technology and the areas of information security, compliance and regulation that need to be considered. Being able to assist with a governance model and human exception-handling framework that maximises accuracy and value and minimises risk would be advantageous. That means your integration with Large Language Models (LLMs) and a personalised model risk management framework will allow your firms to use AI safely and transparently.
You want a controlled gateway that allows your financial services firm to reap the rewards of artificial intelligence and large language models, without the associated risks – meaning you don’t need technical experts in every function to make it work.
What is Extractive AI and how does it fit into the financial ecosystem?
If you feel a little confused about the difference between Extractive and Generative AI you’re not alone. It’s complex, and the more people we speak to across businesses, the more we realise there is an information gap.
The first thing to say is that Extractive AI, like a Formula One engine, is specialised. The engine has one role. To go at 100% power all the time.
With Extraction AI, it’s the same. It performs with precision all the time. Accuracy is the watchword. There’s no room for ‘hallucinations’ here. There is no possibility of making stuff up.
Extractive AI is a type of precision artificial intelligence that focuses on pulling relevant data and information from unstructured data sources.
Think of an array of data, like receipts, annual reports, board papers, meeting minutes, huge Excel files, or financial regulations. Analysing that variety of text, fonts, tables, and statistics is a problem that needs multi-modal artificial intelligence. It needs a combination of NLP (like GPT-X) with machine vision. It’s unique, bespoke, and highly technical.
Now imagine being able to “chat” with those documents in precise detail. “Wait,” I hear you say, “I thought Chat-GPT4/4o could read documents?”
It can. Generative AI can be used for analysis but it has severe limitations, especially when we’re talking about the task of drawing insights from highly specialised data at the immense scale and quantity held, and produced, by global financial services institutions.
Generative AI is a tool to be used in conjunction with a cutting-edge IDP system, not in place of it. You need a high-end Extractive AI solution to make your Generative AI solution work.
Extractive AI | Generative AI | |
Speed to get the right answer | Takes longer but is correct. | Quicker but might be incorrect. |
Accuracy of answers | Strong accuracy. | Not guaranteed. |
Suitability for regulated industries | Suitable. | Not suitable. |
Creative/fun things | Limited. | Unlimited. |
Prompt effort | Precision required. | Less precision required. |
Tolerance for hallucinations/errors | No tolerance. | Allowances should be made for. |
Insert sensitive information into the prompt or model | Encouraged with the right risk framework. | Best avoided unless risk tolerance is acceptable. |
Consistency | Based on data = consistent. | Based on probability = inconsistent. |
There’s a new space race to bring effective enterprise AI into the workplace
Right now there isn’t a company in the world that isn’t reorienting itself towards, or at minimum exploring, the new and powerful opportunities available from artificial intelligence. How do you catch up and stay relevant?
Within financial services and big banks in particular, there is a ready-made use case for an intelligent data processing solution that you can quickly and confidently ask questions of. Let’s move beyond the theoretical and talk about use cases that could already work for you.
For most, the 2008/2009 global financial crisis is still the high water mark for chaos. It’s debatable whether any other event has in and of itself triggered so much new regulation.
The most revealing information about the imminent collapse in US housing lay buried, dormant, and hidden in huge legal contracts that even the lawyers who drafted them didn’t fully understand what was in them.
If you’re a global investment bank with hundreds of billions – or perhaps a trillion – dollars on your balance sheet, you have tens of thousands of these contracts. What if a regulator today wanted to know the details to know exactly what liabilities exist in your financial contracts, and for you to tell them exactly what was in them?
This means going through the text of these cinder block-sized legal documents to pull out small bits of relevant pieces of information from each one before compiling them into something more digestible.
Traditionally, most banks would bring in an army of junior lawyers to sift through piles and piles of documents in a tedious, repetitive manual task that is unconscionably expensive to achieve. You no longer need to do that.
With the right natural language processing and machine learning capabilities, you’d be able to tell them within several hours the exact risk profile of every contract simply by asking the LLM with a simple prompt. And because it was all underpinned by a precise Extractive AI, you could be above 95% certain of the results.